报告题目:Statistical learning and inverse problems from interacting particle systems
报告时间:2023-08-11 15:30-16:30
报告人:Prof. Fei Lu ,Johns Hopkins University
报告地点:理学院东北楼四楼报告厅
Abstract: Systems of self-interacting
particles/agents arise in multiple disciplines, such as particle systems in
physics, flocking birds and migrating cells in biology, and opinion dynamics in
social science. An essential task in these applications is to infer the rules
of interaction from data. We propose nonparametric regression algorithms to
learn the pairwise interaction kernels from trajectory data of differential
systems, including ODEs/SDEs and mean-field PDEs. Importantly, we provide a
systematic learning theory addressing the fundamental issues, such as
identifiability and convergence of the estimators. The algorithms and theory
are demonstrated in examples including opinion dynamics, the Lennard-Jones
system, and aggregation diffusions. Furthermore, learning kernels in operators
emerges as a new topic at the intersection of statistical learning and inverse
problems. We introduce a data-adaptive RKHS Tikhonov regularization (DARTR)
method to address the ill-posednessand discuss open questions on this new topic.
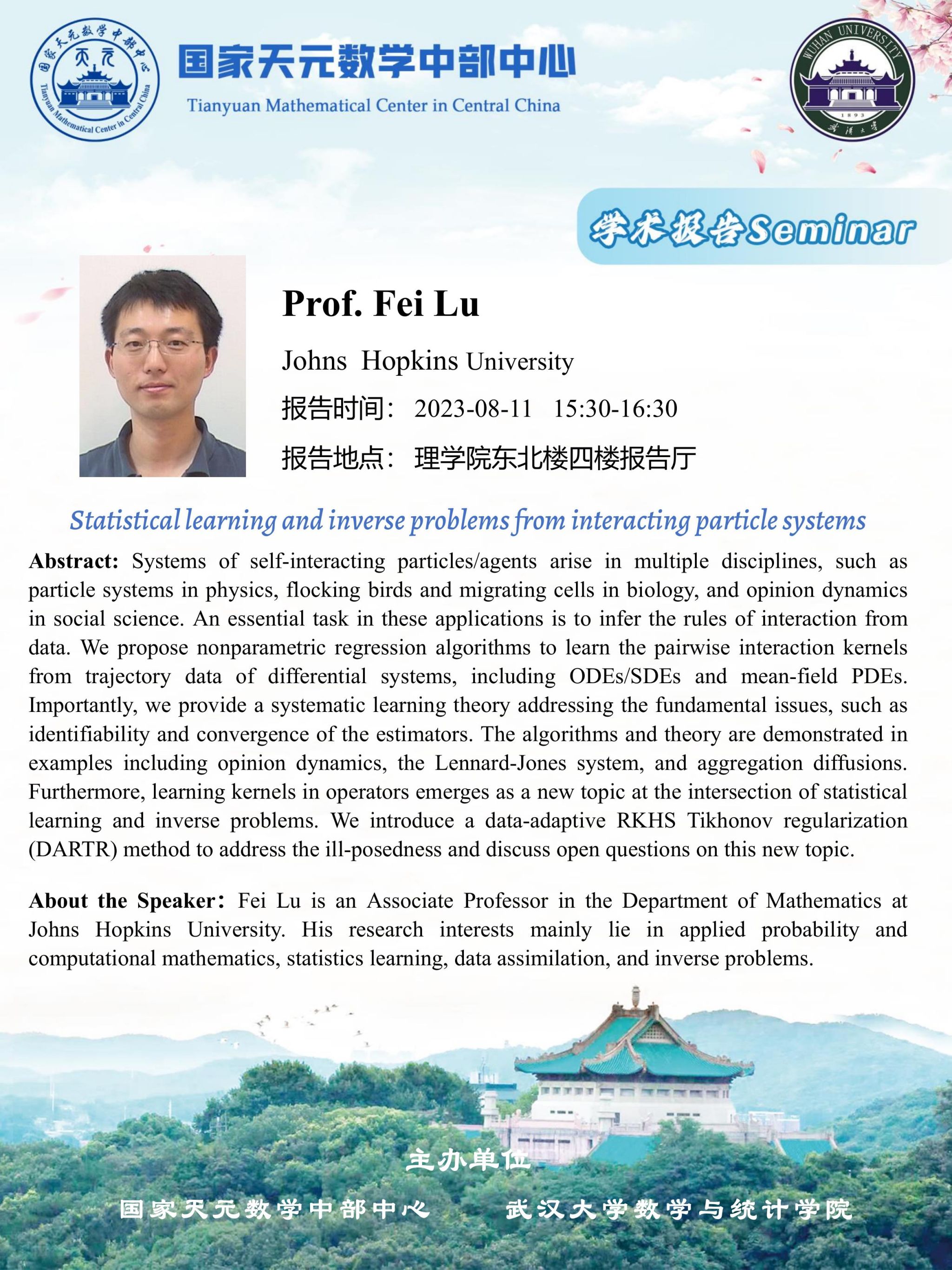