报告题目:Minimax Optimal Learning: Adaptivity, Model Compression and Limitation
报告时间:2023-01-13 10:00 - 11:30
报告人:Prof.Yuhong Yang University of Minnesota
ZOOMID:951 7700 6570 密码:20230113
Abstract:Minimax-rate optimality plays a foundational role in theory of statistical and machine leaning. Besides the identification of minimax-rates of convergence and optimal learning procedures for various learning scenarios, adaptive strategies have also been devised to work simultaneously well for multiple or even infinitely (countably or continuously) many possible scenarios that may describe the underlying distribution of the data. Going with the exciting successes of the modern regression learning tools are questions/concerns/doubts on sparsity, model compressibility, instability, robustness and reliability of the fancy automated algorithms. In this talk, we will first present on minimax optimal adaptive estimations for high-dimensional regression learning under hard and soft sparsity setups, taking advantage of recent sharp sparse linear approximation bounds. An application on model compression in neural network learning will be given. Then we will address the question that how adaptive and powerful any learning procedure really can be. We show that every procedure, no matter how it is constructed, can only work well for a limited set of regression functions.
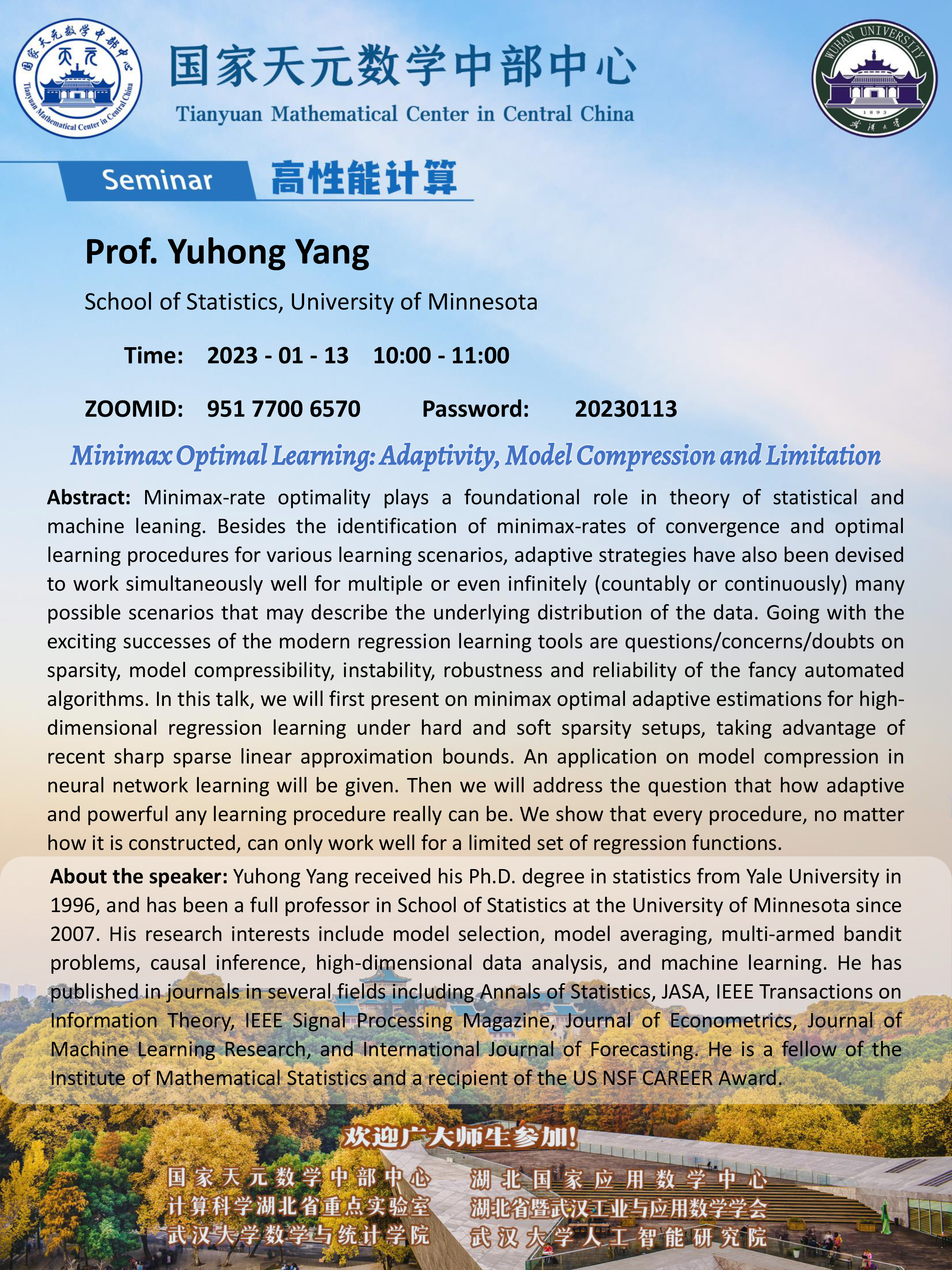