报告题目:Inference and Uncertainty Quantification for Nonconvex Low-Rank Models
报告时间:2022-12-02 09:30 - 10:30
报告人:Prof. Yuxin Chen University of Pennsylvania
腾讯会议ID:828-391-627
报告链接:https://meeting.tencent.com/dm/2OXytd62Rpaj
Abstract:Many high-dimensional problems involve reconstruction of a low-rank matrix from incomplete and corrupted observations. Despite substantial progress in designing efficient estimation algorithms, it remains largely unclear how to assess the uncertainty of the obtained low-rank estimates, and how to construct valid yet short confidence intervals for the unknown low-rank matrix. In this talk, I will discuss how to perform inference and uncertainty quantification for two examples of low-rank models: (1) heteroskedastic PCA with missing data, and (2) noisy matrix completion. For both problems, we identify statistically efficient estimators that admit non-asymptotic distributional characterizations, which in turn enable optimal construction of confidence intervals for, say, the unseen entries of the low-rank matrix of interest. All this is accomplished by a powerful leave-one-out analysis framework that originated from probability and random matrix theory.
This is based on joint work with Yuling Yan, Cong Ma, and Jianqing Fan. See arXiv:2107.12365 and arXiv:1906.04159.
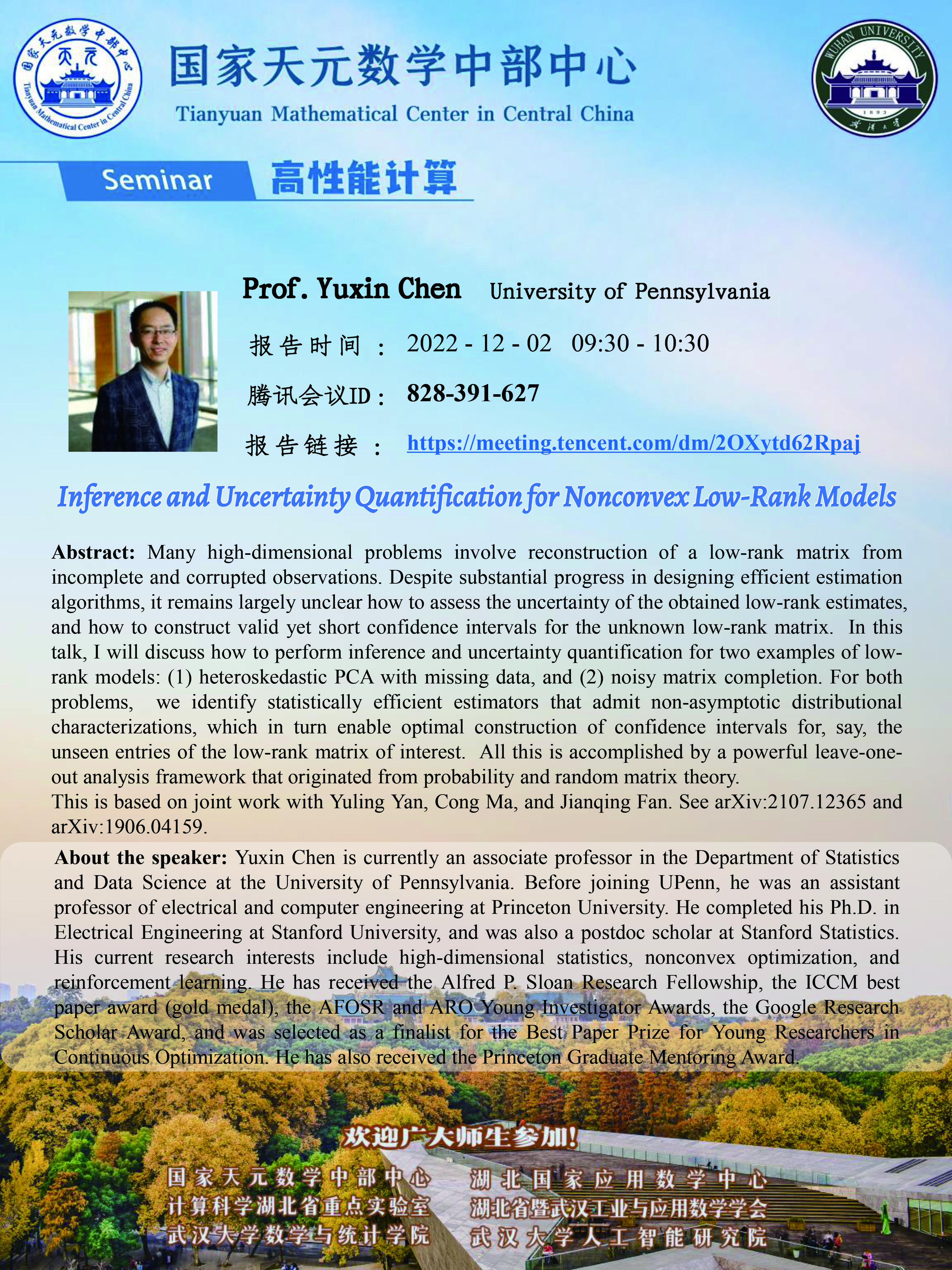