报告题目:Mendelian Randomization for Causal Inference Accounting for Pleiotropy and Sample Structure Using Genome-Wide Summary Statistics
报告时间:2022-03-31 09:50 - 10:30
报告人:杨灿 香港科技大学
ZOOMID:993 6445 5975 密码:220331
报告入口:https://zoom.us/j/99364455975?pwd=VUlvalBWcUtvc3M0aU1IbzJYUUdHUT09
Abstract:Inferring the causal relationship between a risk factor (exposure) and a complex trait of interest (outcome) is essential in biomedical research and social science. Mendelian Randomization (MR) is a valuable tool for inferring causal relationships among a wide range of traits using summary statistics from genome-wide association studies (GWASs). Existing summary-level MR methods often rely on strong assumptions, resulting in many false positive findings. To relax MR assumptions, ongoing research has been primarily focused on accounting for confounding due to pleiotropy. Here we show that sample structure is another major confounding factor, including population stratification, cryptic relatedness, and sample overlap. We propose a unified MR approach, MR-APSS, which (i) accounts for pleiotropy and sample structure simultaneously by leveraging genome-wide information; (ii) allows to include more genetic instruments with moderate effects to improve statistical power without inflating type I errors. We first evaluated MR-APSS using comprehensive simulations and negative controls, and then applied MR-APSS to study the causal relationships among a collection of diverse complex traits. The results suggest that MR-APSS can better identify plausible causal relationships with high reliability. In particular, MR-APSS can perform well for highly polygenic traits, such as psychiatric disorders and social traits, where the strengths of IVs tend to be relatively weak and existing summary-level MR methods for causal inference are vulnerable to confounding effects. This is a joint work with Xianghong Hu, Jia Zhao, Zhixiang Lin, Yang Wang, Heng Peng, Hongyu Zhao, and Xiang Wan.
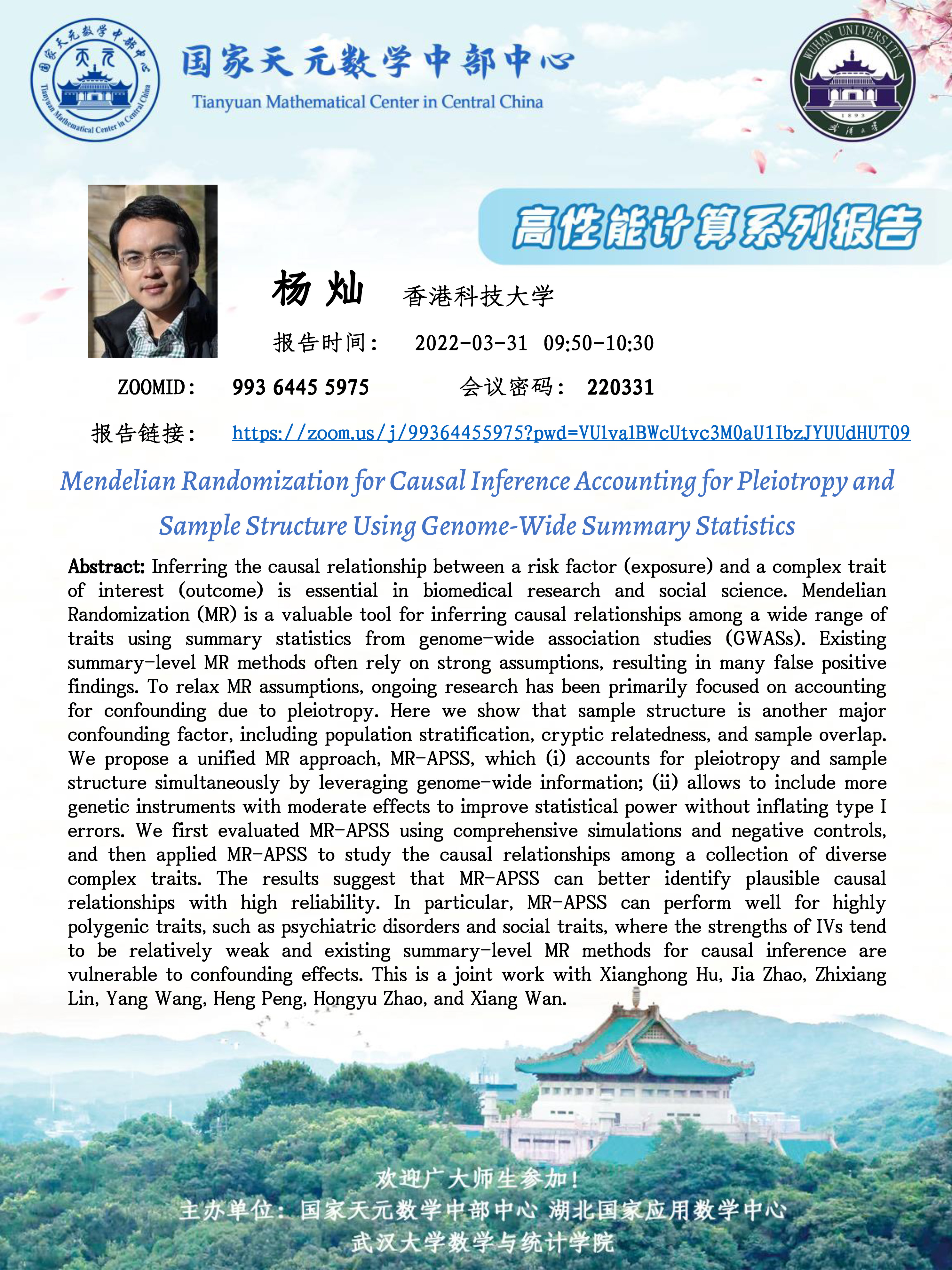