报告题目:Energy Based Models and Adversarial Training
报告时间:2021-12-21 10:00 - 11:00
报告人:孙嘉城 华为诺亚方舟实验室
报告地点:理学院东北楼209
Abstract:Recently, some works found an interesting phenomenon that adversarially robust classifiers can generate good images comparable to generative models. We investigate this phenomenon from an energy perspective and provide a novel explanation. We reformulate adversarial example generation, adversarial training, and image generation in terms of an energy function. We find that adversarial training contributes to obtaining an energy function that is flat and has low energy around the real data, which is the key for generative capability. Based on our new understanding, we further propose a better adversarial training method, Joint Energy Adversarial Training (JEAT), which can generate high-quality images and achieve new state-of-the-art robustness under a wide range of attacks.
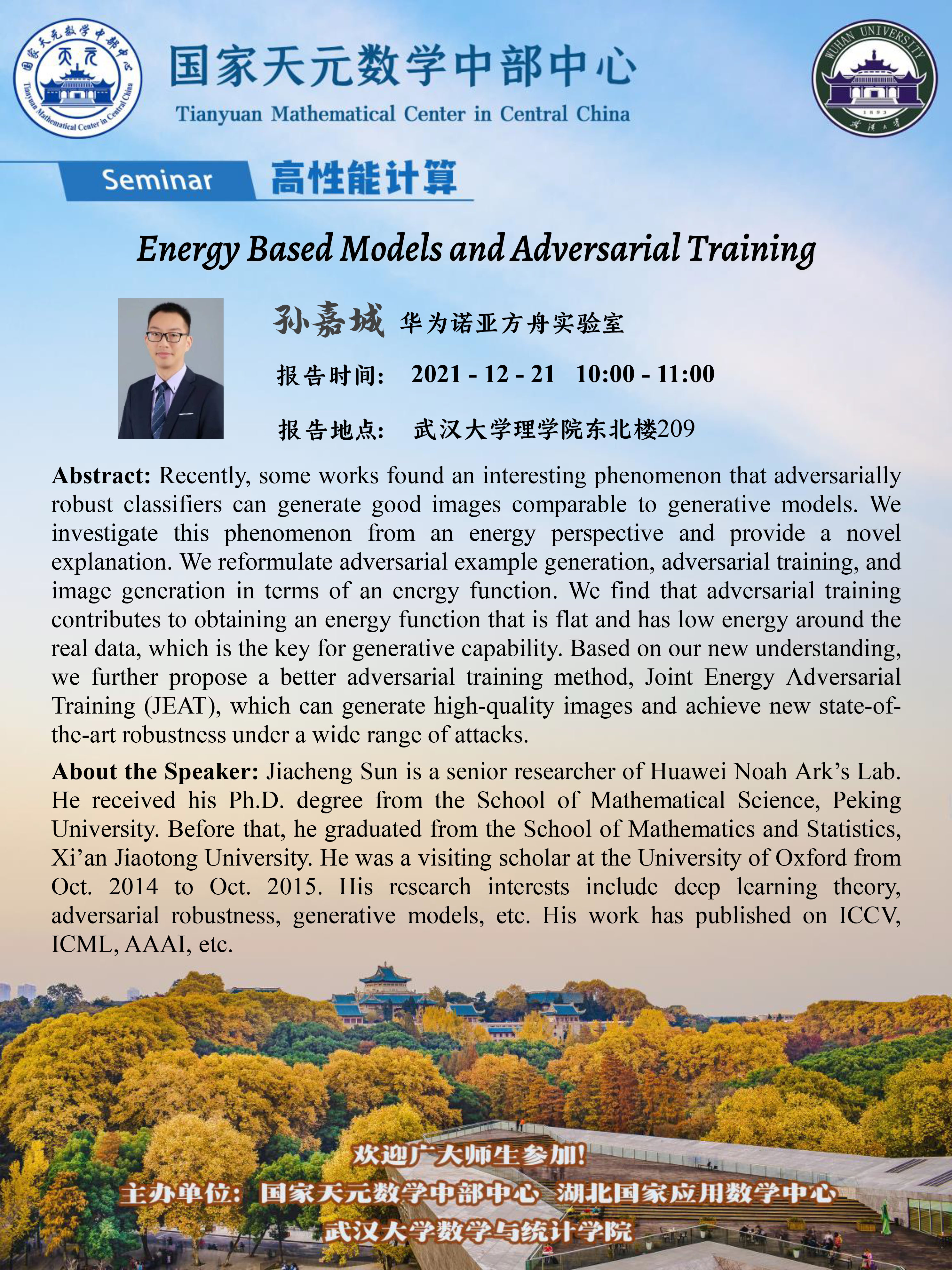