报告题目:Effective Snapshot Compressive-spectral Imaging via Deep Denoising and Total Variation Priors
报告时间:2021-06-05 09:50 - 10:40
报告人:王尧 教授 西安交通大学
报告地点:武汉大学理学院东北楼404
主办单位:国家天元数学中部中心 武汉大学数学与统计学院
Abstract : Snapshot compressive imaging (SCI) is a new type of compressive imaging system that compresses multiple frames of images into a single snapshot measurement, which enjoys low cost, low bandwidth, and high-speed sensing rate. By applying the existing SCI methods to deal with hyperspectral images, however, could not fully exploit the underlying structures, and thereby demonstrate unsatisfactory reconstruction performance. To remedy such issue, this work aims to propose a new effective method by taking advantages of two intrinsic priors of the hyperspectral images, namely deep image denoising and total variation (TV) priors. Specifically, we propose an optimization objective to utilize these two priors. By solving this optimization objective, our method is equivalent to incorporate a weighted FFDNet and a 2DTV or 3DTV denoiser into the plug-and-play framework. Extensive numerical experiments demonstrate the outperformance of the proposed method over several state-of-the-art alternatives. Additionally, we provide a detailed convergence analysis of the resulting plug-and-play algorithm under certain conditions.
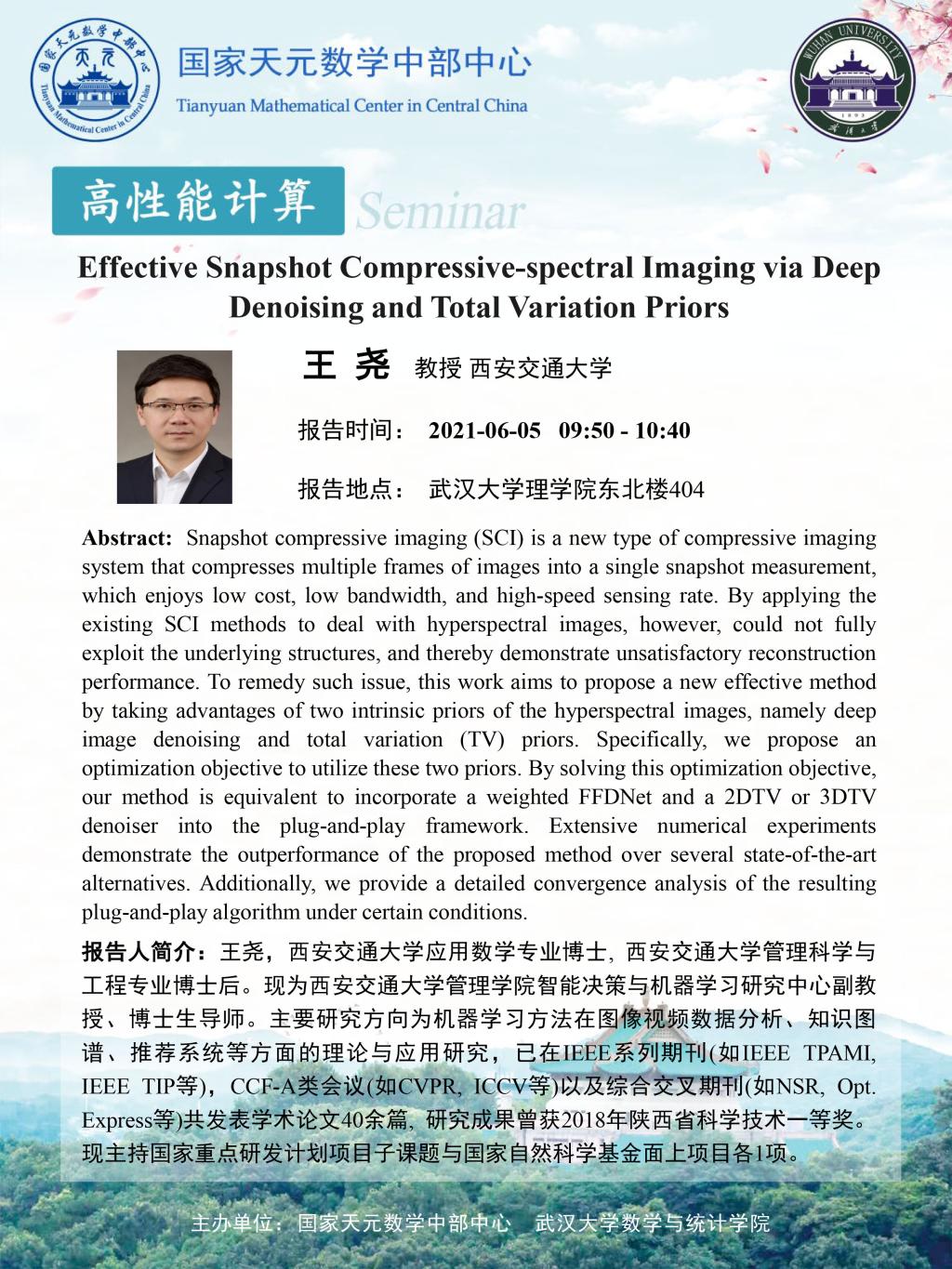