2021年计算神经科学系列报告(四)--计算模型和可扩展的方法用于大规模神经数据分析
Computational Models and Scalable Methods for Large-Scale Neural Data Analysis
报告时间:2021-05-27 20:00 - 21:00
报告人:陈哲 纽约大学
会议地点:zoom会议室
ID:659 0603 6570 密码:20210527
主办单位:国家天元数学中部中心
组委会:黄橙橙 (匹兹堡大学), 张继伟 (武汉大学), 周栋焯 (上海交通大学)
Abstract: Advances in neurotechnologies have allowed us to record large-scale neural signals from electrophysiology and calcium imaging. However, current exponential growth in the scale of data acquisition also presents an analysis challenge. I will first discuss the trend and bottleneck of statistical analysis for high-dimensional neural data, touching the issue of dimensionality reduction, subsampling, adaptive closed-loop experimental design. Finally, I will use the rodent hippocampus as an example to illustrate the development of scalable methods for analyzing large-scale hippocampal population codes based on multimodal recordings (spikes, local field potentials and calcium imaging).
A few reading materials:
Chen SZ, Pesaran B (2021). Improving scalability in systems neuroscience. Neuron, 109,
https://doi.org/10.1016/j.neuron.2021.03.025.
Cao L, Varga V, Chen SZ (2021). Spatiotemporal patterns of rodent hippocampal field potentials uncover spatial representations.
http://biorxiv.org/cgi/content/short/828467v1
Tu M, Zhao R, Adler A, Gan WB, Chen SZ (2020). Efficient position decoding methods based on fluorescence calcium imaging in the mouse hippocampus. Neural Computation, 32(6): 1144-1167.
Hu S, Ciliberti D, Grosmark AD, Michon F, Ji D, Buzsaki G, Wilson MA, Kloosterman F, Chen SZ (2018). Real-time read out for large-scale unsorted neural ensemble place codes. Cell Reports, 25: 2635-2642.
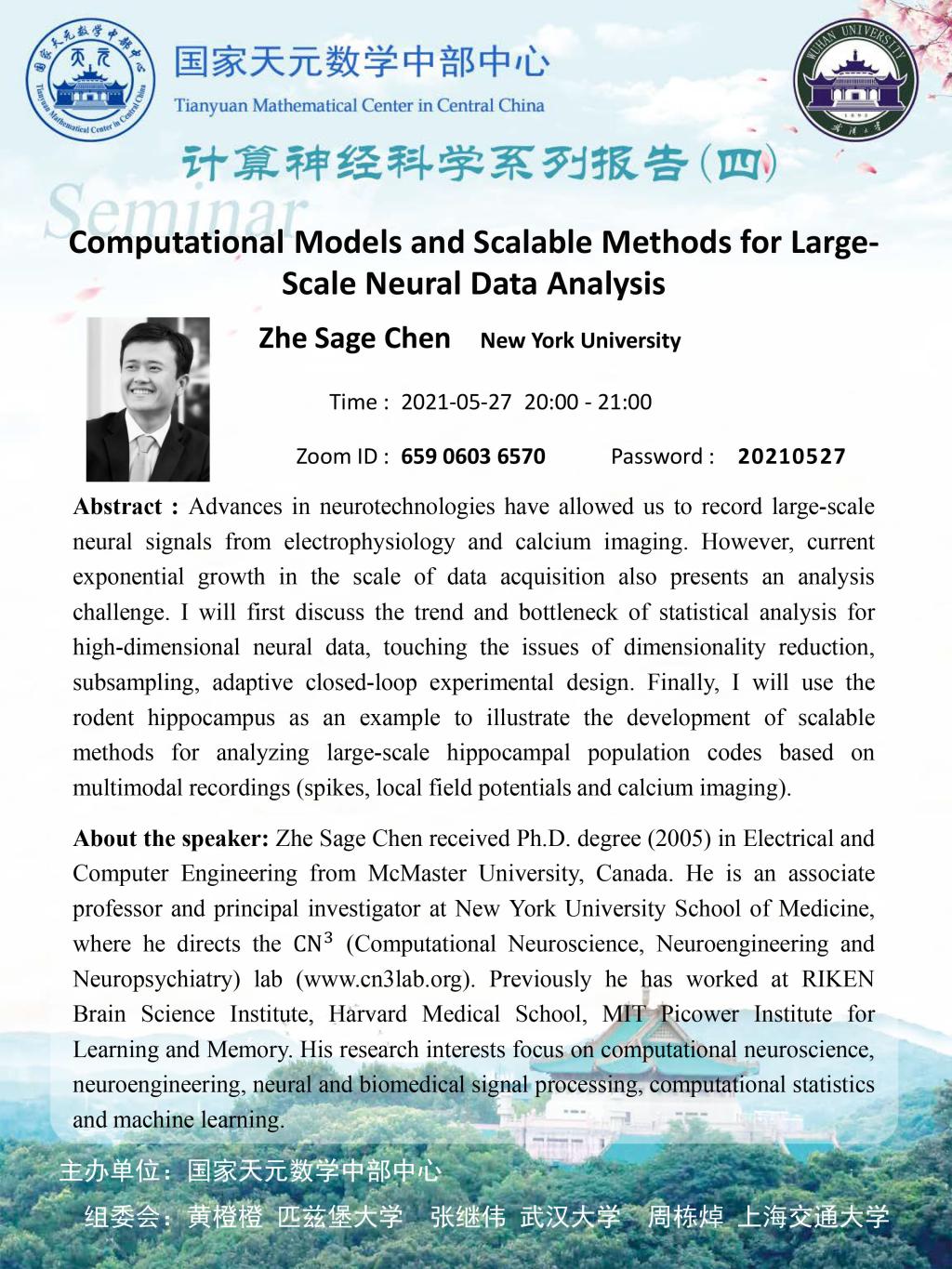