报告题目:Theoretical Insights into Self-Supervised Contrastive Learning
报告时间:2024-05-15 10:00-11:00
报 告 人:黄维然 副教授(上海交通大学)
腾讯会议ID:784 515 754
Abstract:Self-supervised learning has recently
attracted significant attention because it requires only unlabeled data for
model training. Contrastive learning, a popular method within self-supervised
learning, has demonstrated promising empirical performance. However, the
theoretical understanding of its generalization ability remains limited. In
this talk, I will analyze self-supervised contrastive learning from a
theoretical perspective, demonstrating that its generalization ability is
linked to three key factors: the alignment of positive samples, the divergence
of class centers, and the concentration of augmented data. Moreover, I will show that self-supervised
contrastive learning fails to learn domain-invariant features, thereby limiting
its transferability. To address this issue, I will introduce
Augmentation-robust Contrastive Learning (ArCL)
and show how it significantly improves the transferability of self-supervised
contrastive learning.
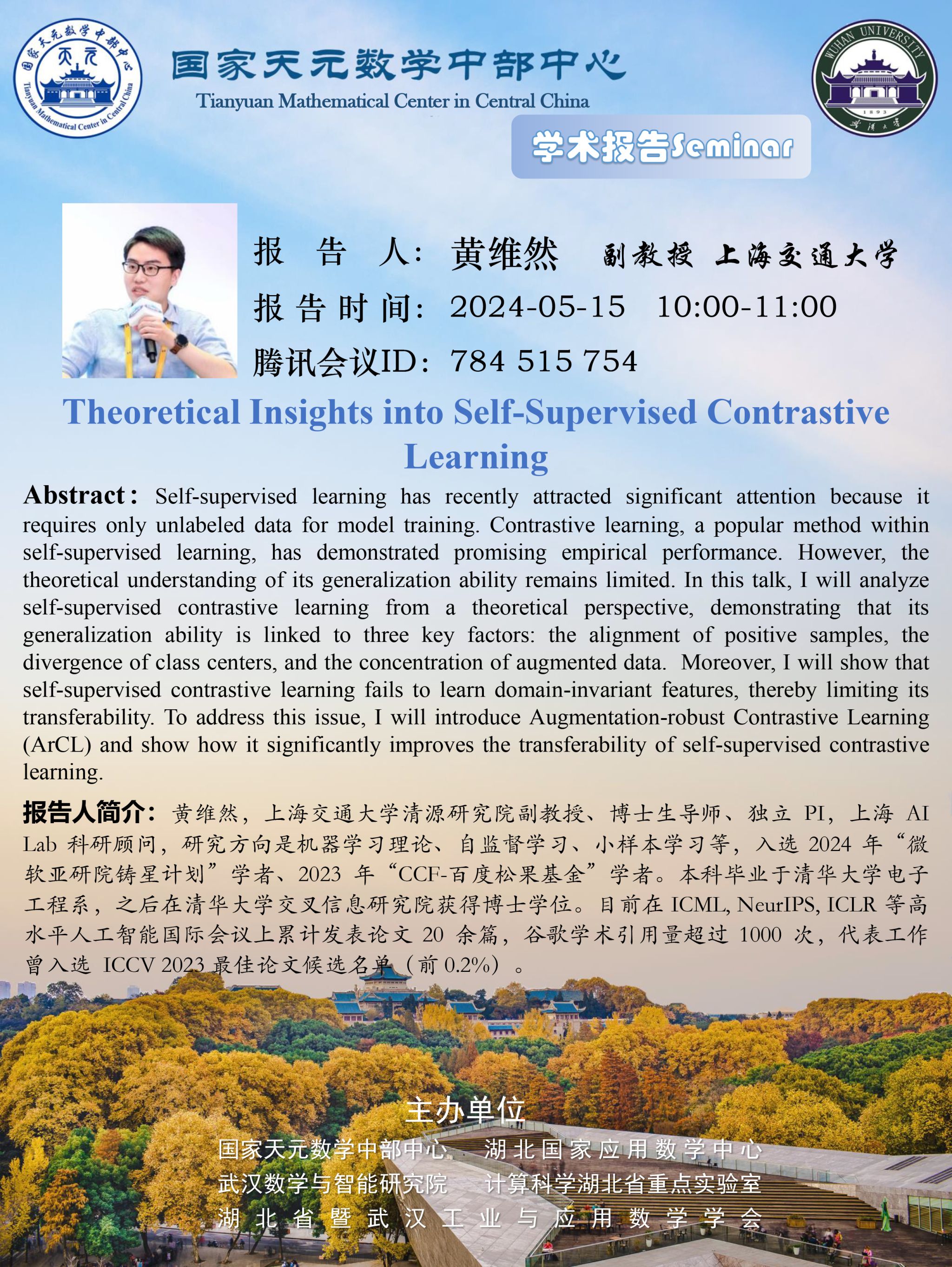