报告题目:高维成分数据统计分析理论方法
报告时间:2024-06-12 15:30-17:00
报 告 人:占翔 副教授(北京大学)
报告地点:理学院东北楼四楼报告厅(404)
报告摘要: It is quite common to encounter
compositional data in modern data sciences. Most existing statistical methods
for compositional data analysis are based on a log-ratio transformation that
moves compositional data analysis from simplex to reals. Under this framework,
we first investigate novel statistical methods for reliable and reproducible
variable selection analysis on compositional predictors. The second part of
this talk is about composition-on-composition regression. When both responses
and predictors are compositional, the inventory of statistical analysis tools
is surprisingly limited. Motivated by data analysis problems with
high-dimensional microbiome compositional data, we propose the
Composition-On-Composition (COC) regression analysis, which does not require
log-ratio transformations and hence can handle excessive zeroes in microbiome
data. We introduce a penalized estimation equation approach in COC to improve
its estimation accuracy in high-dimensional settings and then establish inference
procedures to quantify uncertainties in model estimation and prediction. The
proposed methods are evaluated using both numerical simulations and real data
applications to demonstrate its validity and superiority.
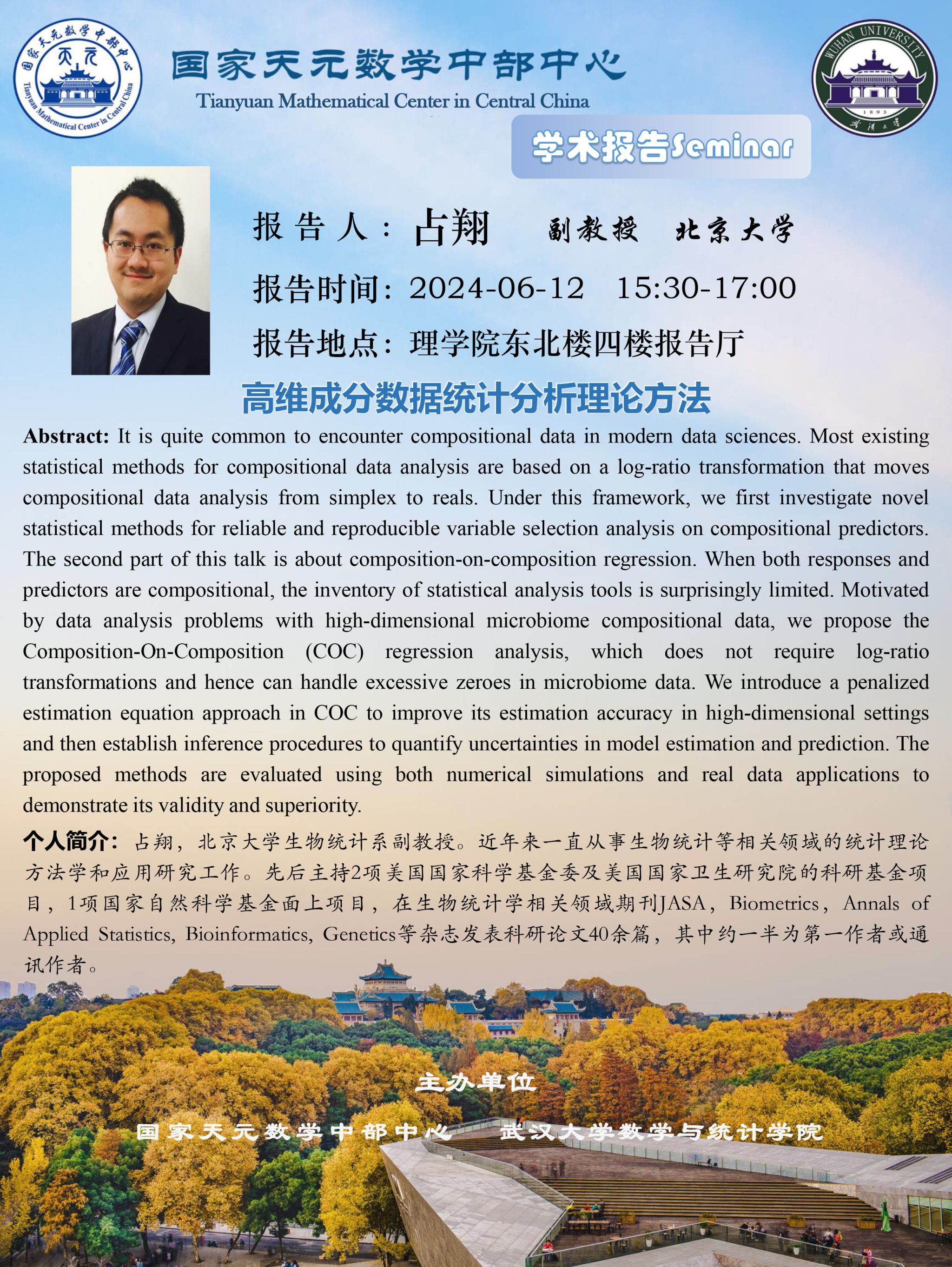