报告题目:基于多步凸优化的高维稀疏指数追踪
报告时间:2024-06-21 10:00-11:30
报 告 人:舒连杰 教授 (澳门大学)
报告地点:腾讯会议 991-493-307
报告摘要: For financial index tracking, a
sparse tracking portfolio with only a small number of assets is often desirable
in practice to avoid small and illiquid positions and large transaction costs.
Owing to its computational efficiency and variable selection properties, the
regularization technique based on the least absolute shrinkage and selection
operator (LASSO) has been widely discussed for high-dimensional sparse index
tracking. However, a relatively large regularization parameter needs to be
selected in LASSO to generate a very sparse solution. This could lead to
relatively large biases in the estimates of the tracking portfolio weights,
which would in turn deteriorate the out-of-sample tracking performance.
Although non-convex penalties could be used to improve the bias issue of LASSO
penalty, the resulting problem is non-convex optimization, which is often
difficult and computationally intensive. Aimed at countervailing bias while
preserving computational efficiency, this paper proposes a multi-step convex
optimization approach based on the multi-step weighted LASSO (MSW-LASSO) for
sparse index tracking. The proposed approach is very general and nests the
traditional LASSO and its variations as special cases. Empirical results show
that the proposed method can achieve smaller out-of-sample tracking errors than
the classical approaches based on LASSO regularization.
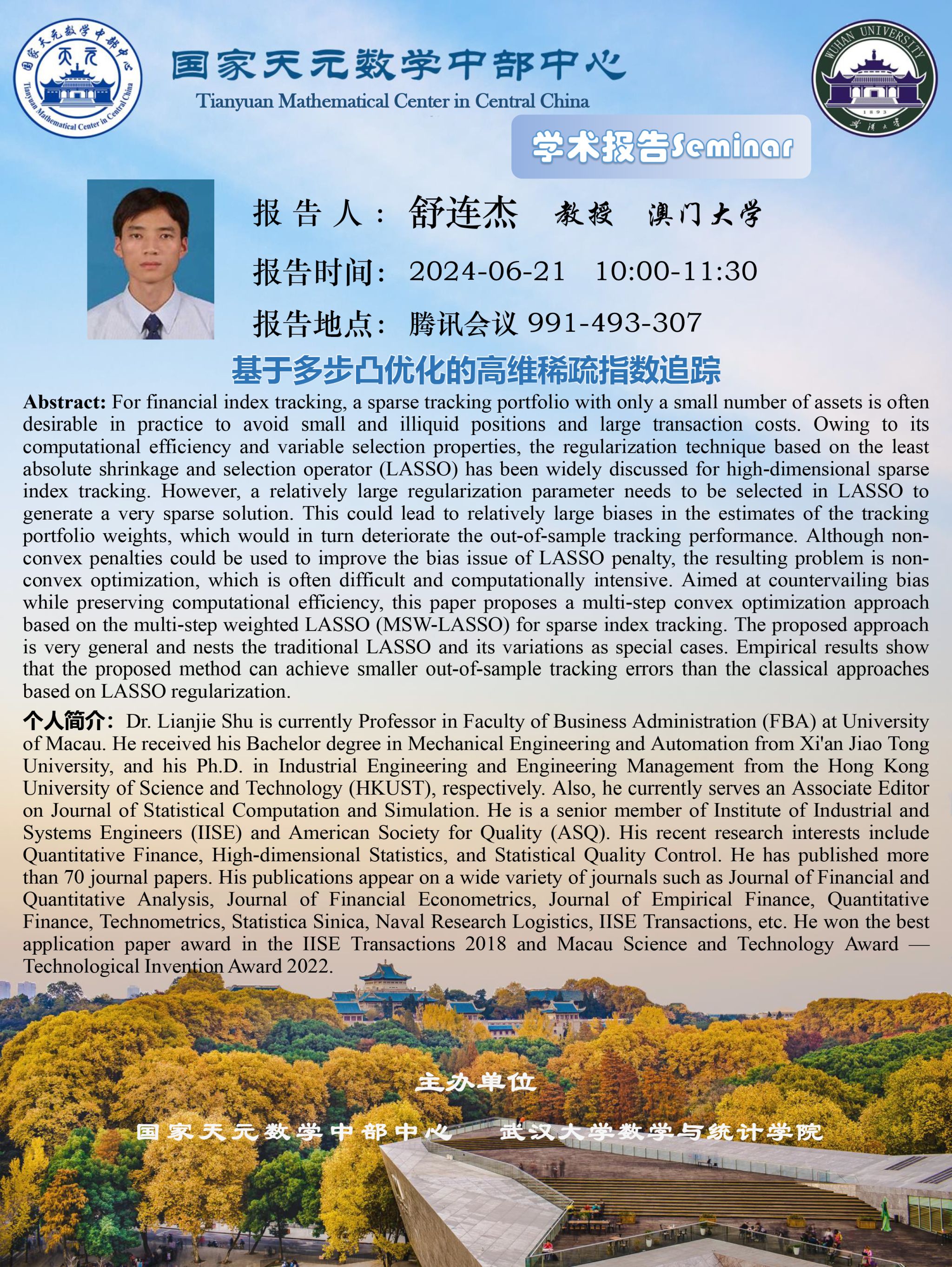