报告题目:Dynamic Tilted Current Correlation for High-Dimensional Variable Screening
报告时间:2024-06-27 16:00-17:00
报 告 人:Prof. Wenqing He (University of Western Ontario,Canada)
报告地点:理学院东北楼二楼报告厅(209)
Abstract: High dimensionality brings distorted results and computational burden to statistical analysis of this type of data. In the ultra-high dimensional setting, Sure Independence Screening was introduced to significantly reduce the dimensionality of variables to a moderate scale below the sample size and to preserve the true model with probability tending to 1. The performance of SIS depends on the marginal correlation which is unreliable when the dimension is high. The importance of the variables cannot be easily ranked by their marginal correlations when there are high correlations among predictor variables. Due to the dimensionality, important predictors may have small marginal correlations with the response, while unimportant predictors may be highly correlated with the response variable due to the associated or spurious correlation with the important predictors. To remove those unimportant predictors and keep real important predictors, we propose a new estimator for the correlation between the response and variables in high dimensional settings, and a new screening technique termed dynamic tilted current correlation screening (DTCCS) is employed to do the variable screening. The new method reduces high spurious correlation among predictor variables in a data-driven fashion. We show that DTCCS can discover all relevant predictor variables within a finite number of steps when the dimensional of the true model is finite. DTCCS's sure screening property, consistency property and computational complexity are justified theoretically and numerically. To confirm the effectiveness of the proposed methods, extensive simulation studies are conducted, and a real data analysis is invoked for illustration.
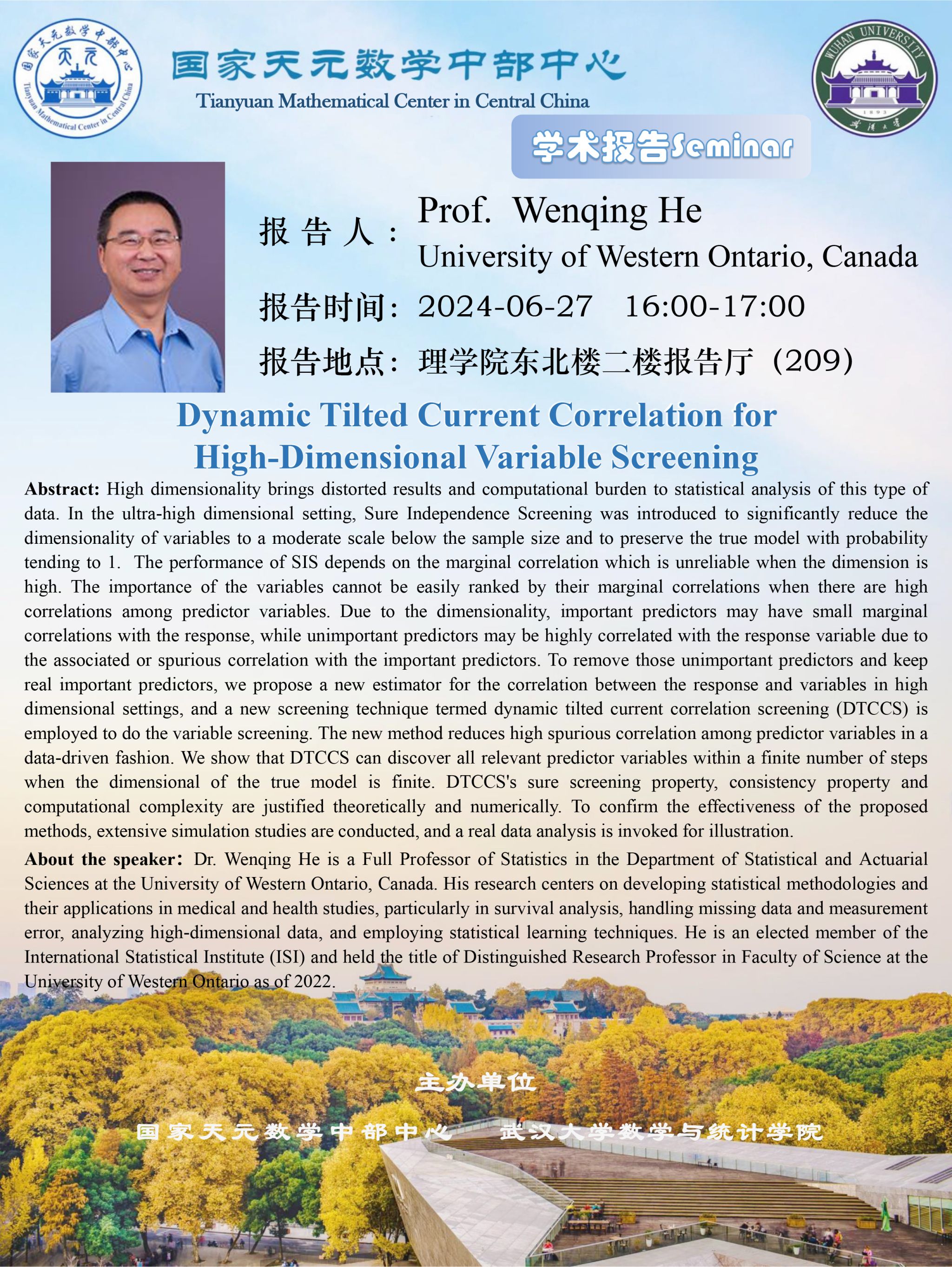