报告题目:必要充分计算机仿真试验打通精准统计推断之路
报告时间:2024-10-17 10:00-11:30
报 告 人:张正军 教授(中国科学院大学)
报告地点:数学院二楼报告厅
Abstract: Modern AI needs accurate
statistical inference methods. The existing statistical estimations were often
derived from some sufficient conditions but not necessary. We first establish a
fundamental theorem that guarantees the transformed order statistics from the
assumed distribution of a random variable (or an error term) to be arbitrarily
close to the order statistics of a simulated sequence of the same distribution.
The theorem leads to a necessary and sufficient condition for two series of
random variables to follow the same distribution. Based on the condition, we
propose a new necessary and sufficient estimation (NSE) method for preserving
continuous distribution assumptions in various statistical studies. Unlike the
Kolmogorov-Smirnov statistics and many other statistics based on absolute
errors between the empirical distribution and the assumed distribution, the
statistics proposed are based on relative errors between the transformed and
simulated order statistics. Surprisingly, relative errors result in much faster
convergence rates than absolute errors. Using the constructed statistic (or the
pivotal quantity in estimation) to measure the relative distance between two
ordered samples, we estimate parameters to minimize the distance. Furthermore,
unlike many existing methods, which rely on some regularity conditions and/or
the explicit forms of probability density functions, the NSE only assumes a
mild condition that the cumulative distribution function can be approximated to
a satisfying precision. The NSE can be directly applied to many kinds of
statistical distribution inference problems regardless of whether existing
estimation methods are applicable. Furthermore, the NSE provides not only point
estimations but also interval estimations to first preserve the model
assumption and then guarantee the significance of parameters. Using NSE,
researchers and practitioners no longer need to assume any moment conditions to
derive asymptotic results. There is no need to conduct the bootstrap method
when the limiting distributions are not computable. This talk illustrates
simulation examples and real applications to show NSE's superior performance
for various commonly applied inference problems where existing estimation
methods may fail to guarantee desired distributional assumptions. Joint work
with BingyanWang (Princeton) and Xinyang Hu (Yale).
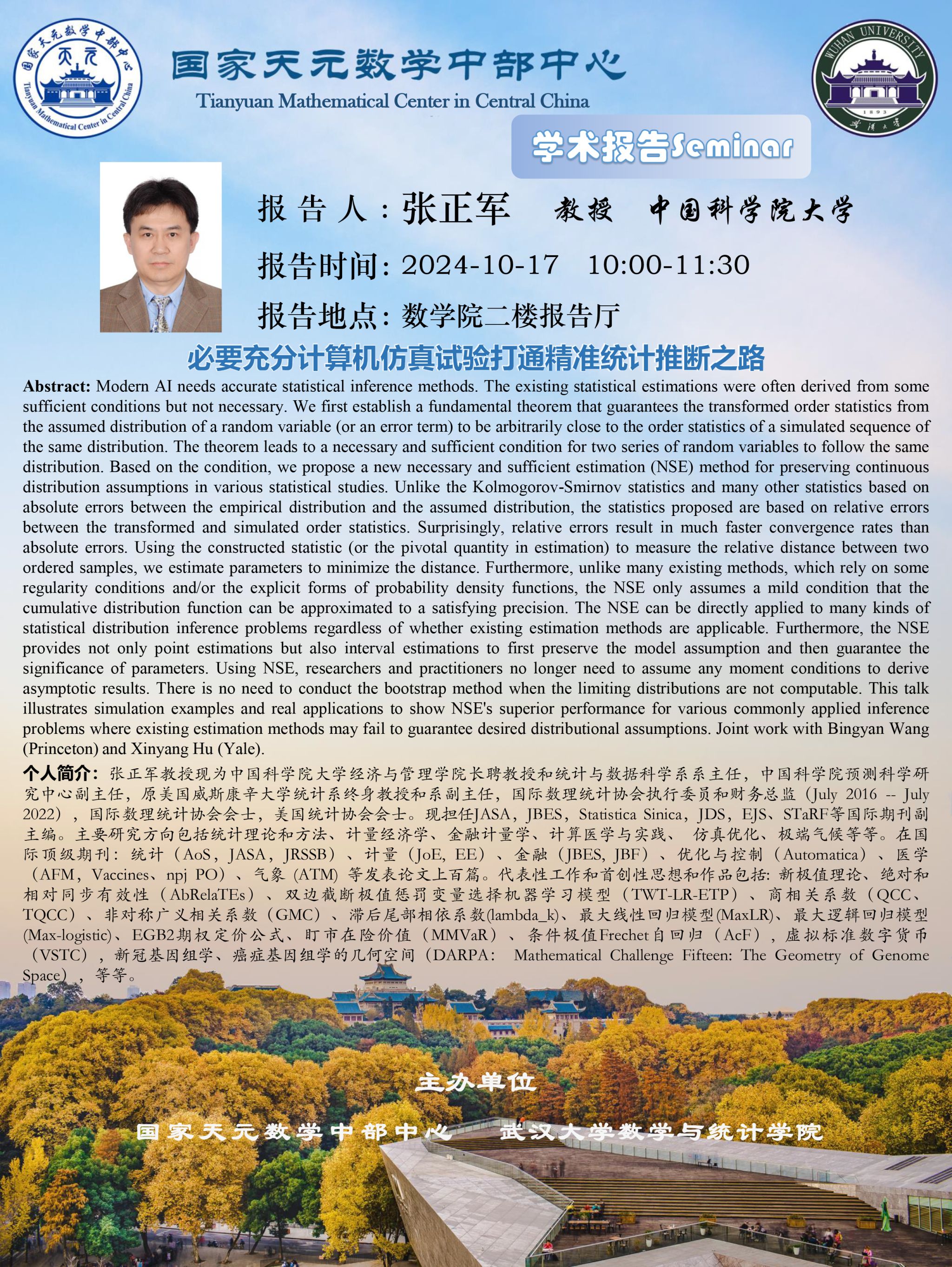