报告题目:Periodicity Learning for Non-stationary Functional Time Series
报告时间:2024-10-24 14:00-15:00
报 告 人:尤进红 教授(上海财经大学)
报告地点:611-957-406(腾讯会议ID)
Abstract: Existing literature on non-stationary functional time series primarily focuses on testing for stationarity and the existence of periodicity or trends. In this article, we present the first attempt to investigate the non-stationary periodic functional time series that has an unknown period and a functional trend. To achieve this, we propose a nonparametric profile estimation method that accurately estimates the unknown period, periodic component, and trend function. We establish the asymptotic properties of the estimators, including period estimate consistency and the asymptotic behaviours of the estimated periodic component and trend function. The finite sample performance of our method is investigated through simulation studies. Additionally, we apply our method to three real data applications, which include the functional time series of global temperature anomaly curves, daily CO and NOX concentrations in Hong Kong, and annual sunspot numbers. We report several intriguing discoveries. For instance, the estimated period of the functional time series of global temperature anomaly curves is 46 years, which is much shorter than the previously estimated period of 60 years based on conventional time series analysis.
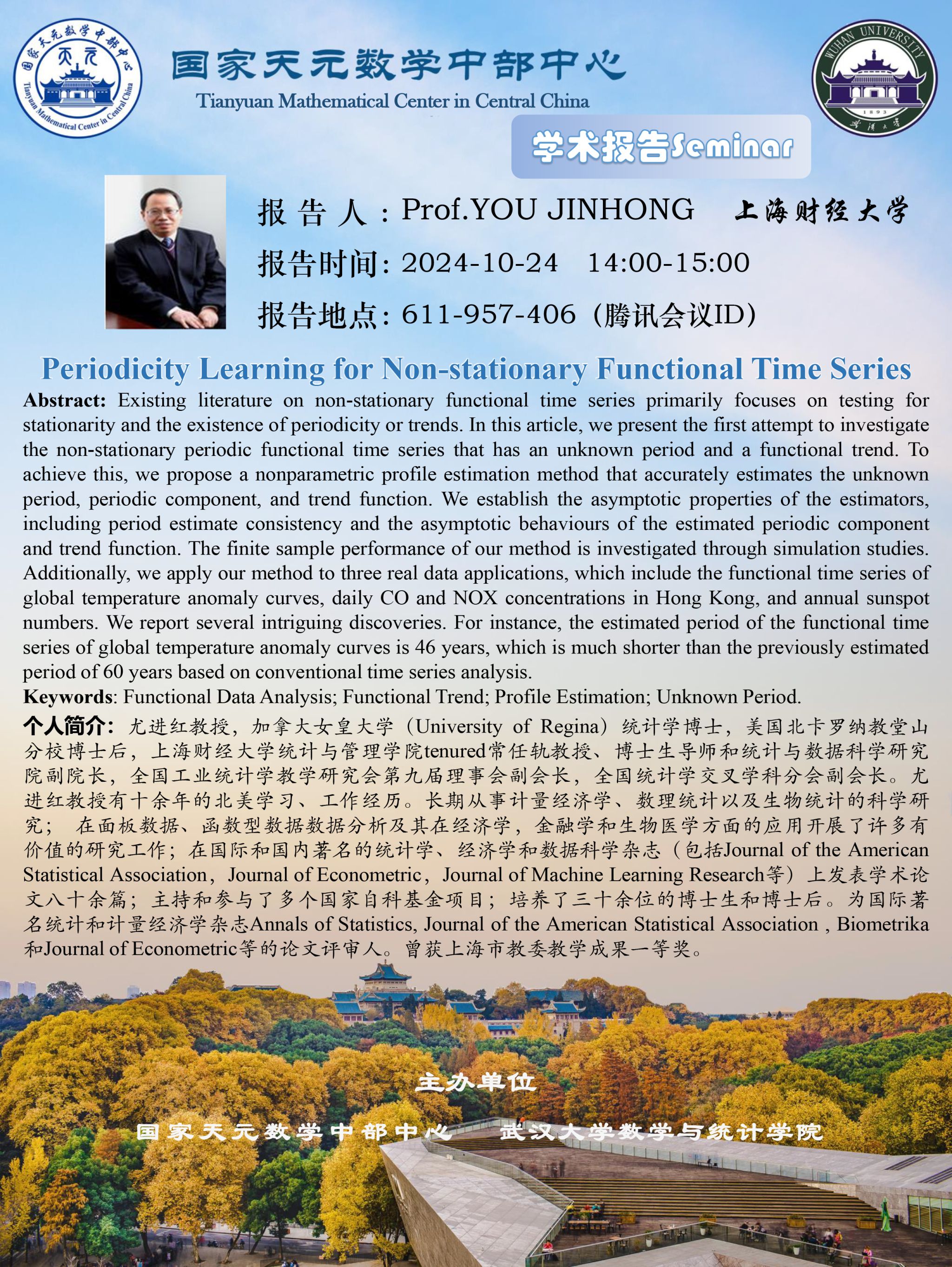