报告题目:Integrating Machine Learning Techniques in Turbulence Closure Models
报告时间:2024-11-16 10:00-11:00
报 告 人:连复桑 教授 (University of Waterloo)
报告地点:老外楼301
Abstract:Industries such as aerospace, automotive,
chemical, nuclear, hydroelectric, and wind power use numerical simulations of
turbulent flows to design safe and efficient systems. However, the inherent
complexity of turbulence equations makes these simulations computationally
demanding. The widely-used Reynolds-averaged Navier-Stokes
(RANS) approach simplifies these equations but often introduces significant
errors due to the eddy viscosity approximation in turbulence closure models.
Despite decades of research, existing turbulence models frequently fail to
capture all relevant industrial physics. Recently, deep learning has emerged as
a promising tool to enhance turbulence closure modeling. Unlike traditional
methods based on intuition and heuristics, machine learning can derive complex
functional relationships from data, potentially overcoming the limitations of
conventional models. This seminar will explore various machine-learning
approaches aimed at improving turbulence closure models. It will focus on an
approach that corrects the Reynolds stress anisotropy tensor—a primary source
of error in RANS models—by training a model to predict high-fidelity closure
terms from low-fidelity inputs. The corrected anisotropy tensor is then
integrated into the momentum equation, resulting in improved mean-field
predictions. However, incorporating machine learning outputs into coupled
partial differential equations demands careful attention to numerical stability
and solution conditioning.
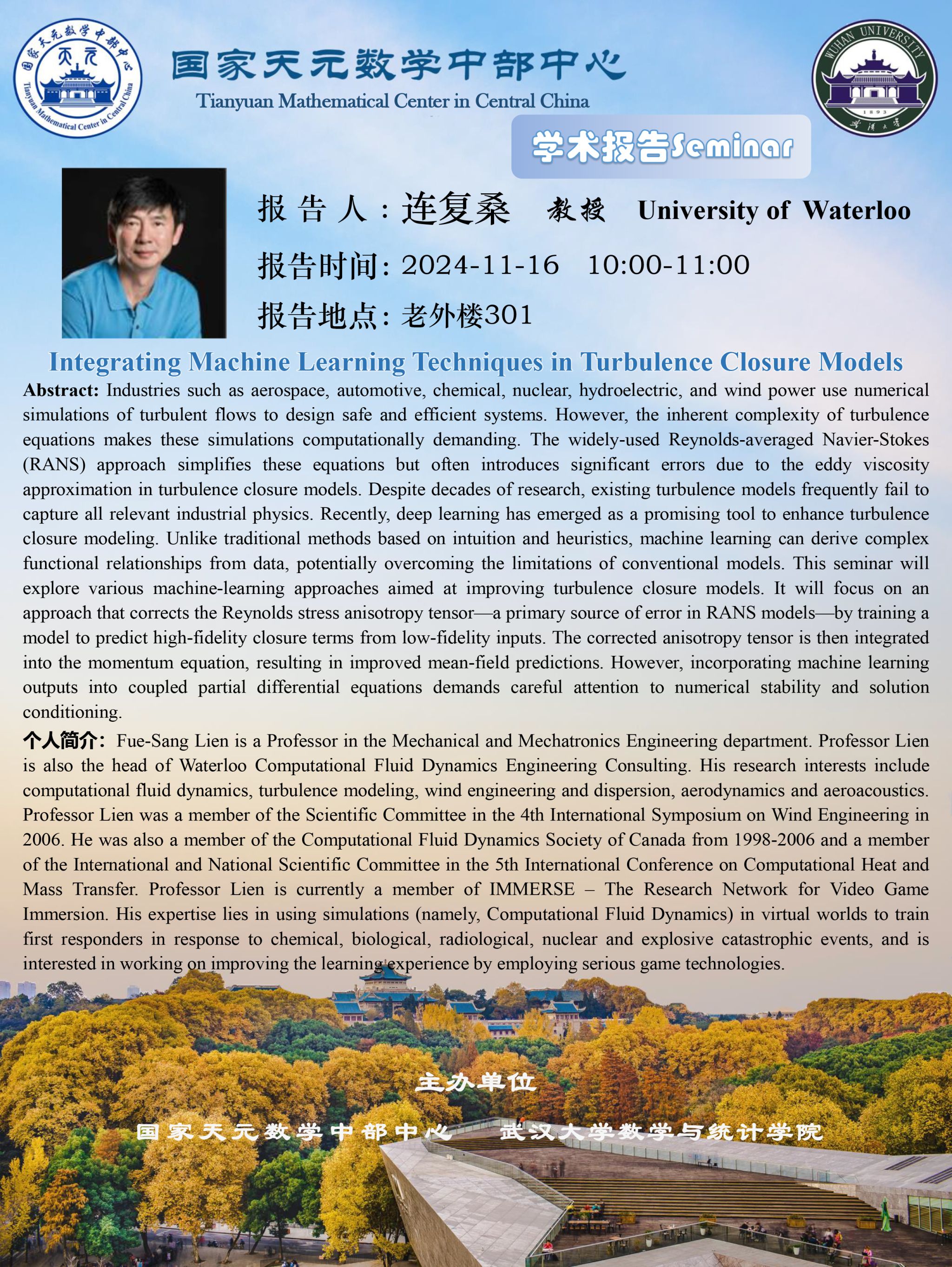