报告题目:RADA: A Flexible Algorithmic Framework for Nonconvex-Linear Minimax Problems on Riemannian Manifolds
报告时间:2025-1-3 10:30-11:30
报 告 人:刘亚锋 副研究员(中国科学院数学与系统科学研究院)
报告地点:雷军科技楼八楼报告厅(806)
Abstract:Recently, there has been growing interest in minimax problems on Riemannian manifolds due to their wide applications in machine learning and signal processing. Although many algorithms have been developed for minimax problems in the Euclidean setting, relatively few works studied minimax problems on manifolds. In this talk, we focus on the nonconvex-linear minimax problem on Riemannian manifolds. We propose a flexible Riemannian alternating descent ascent (RADA) algorithmic framework and prove that it achieves the best-known iteration complexity known to date. Various customized simple yet efficient algorithms can be incorporated within the proposed algorithmic framework and applied to different problem scenarios. We also reveal intriguing connections between the algorithms developed within our proposed framework and existing algorithms, which provide important insights into why the former outperform the latter. Lastly, we report extensive numerical results on sparse principal component analysis (PCA), fair PCA, and sparse spectral clustering to demonstrate the superior performance of the proposed algorithms.
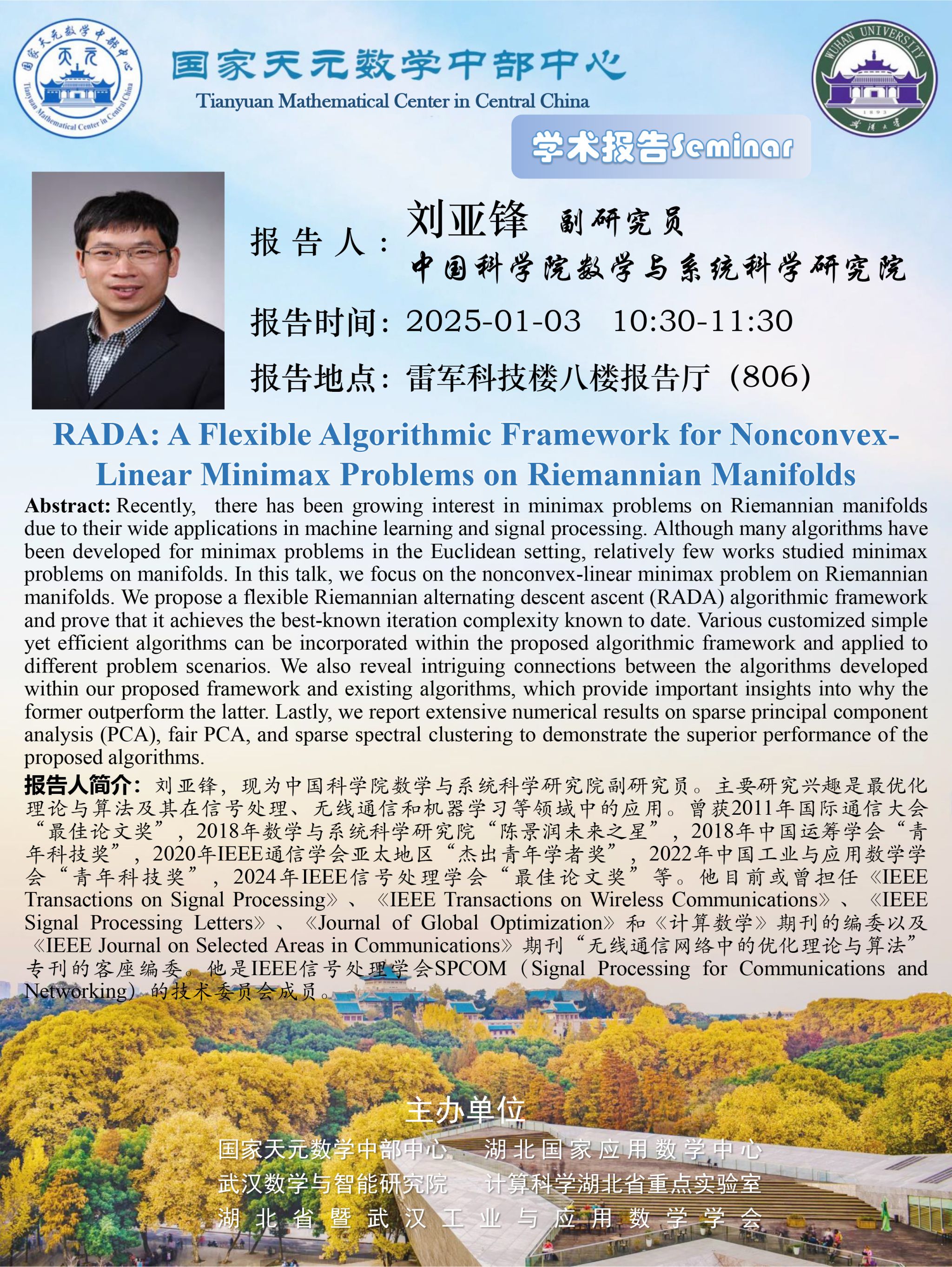