报告题目:Exploring the Optimal Choice for Generative Processes in Diffusion Models
报告时间:2023-12-18 15:00-16:00
报 告 人 :曹语 副教授 上海交通大学
报告地点:理学院东北楼一楼报告厅(110)
Abstract:The diffusion model has shown
remarkable success in computer vision, but it remains unclear whether the
ODE-based probability flow or the SDE-based diffusion model is more superior
and under what circumstances. Comparing the two is challenging due to dependencies
on data distributions, score training, and other numerical issues. In this
talk, we will discuss a mathematical approach for this problem by considering
two limiting scenarios: the zero diffusion (ODE) case and the large diffusion
case. We will demonstrate that the time distribution of the score training
error will determine the optimal dynamics in terms of minimizing the sampling
error in the continuous-time setting. Numerical validation of this phenomenon
is provided using various benchmark distributions, as well as realistic
datasets like MNIST and CIFAR-10. This talk is based on a joint work with JingrunChen, YixinLuo, and Xiang Zhou.
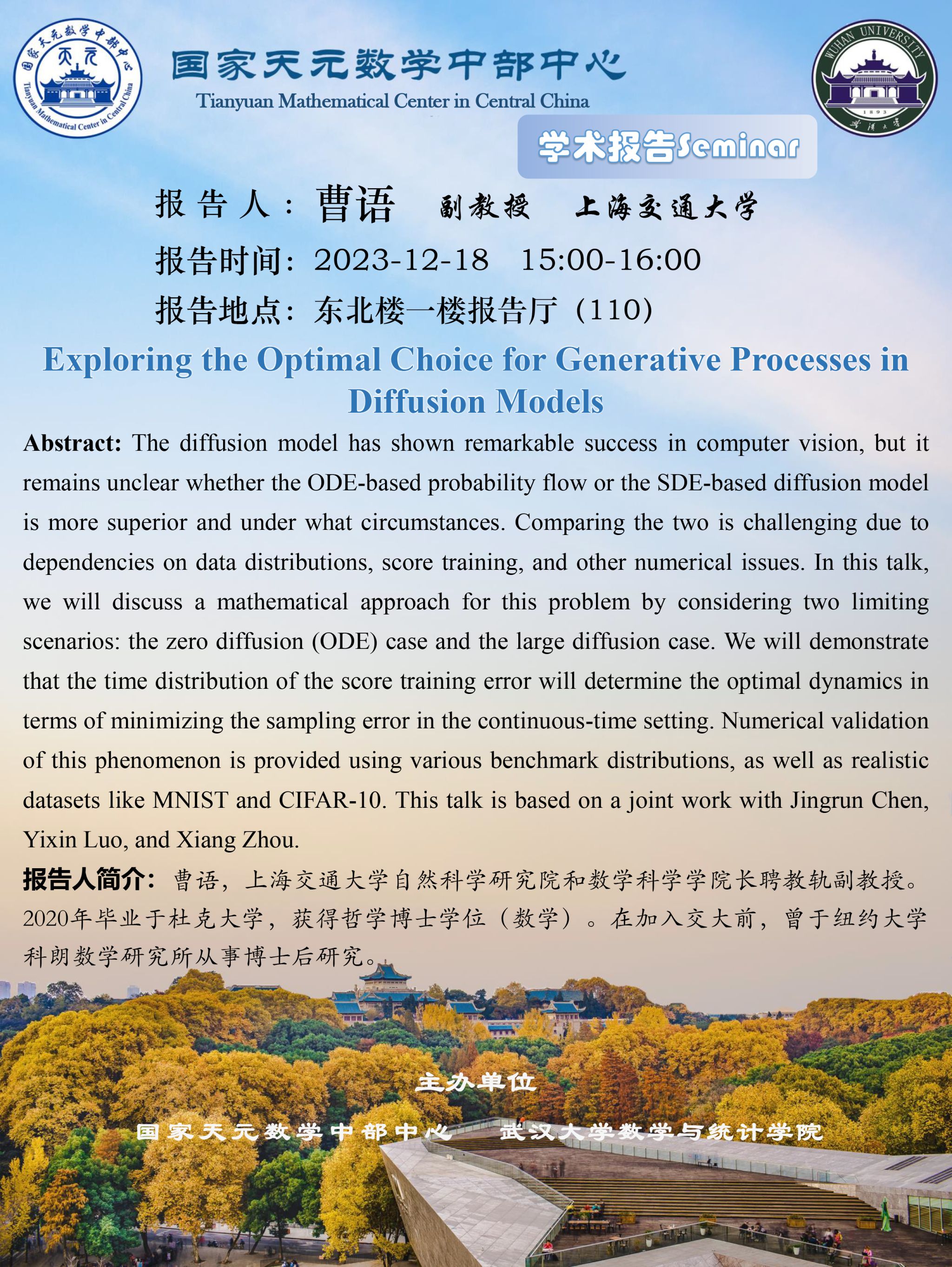